Prediction Of Icing Risk Degree On Aircraft With Machine Learning Algorithms
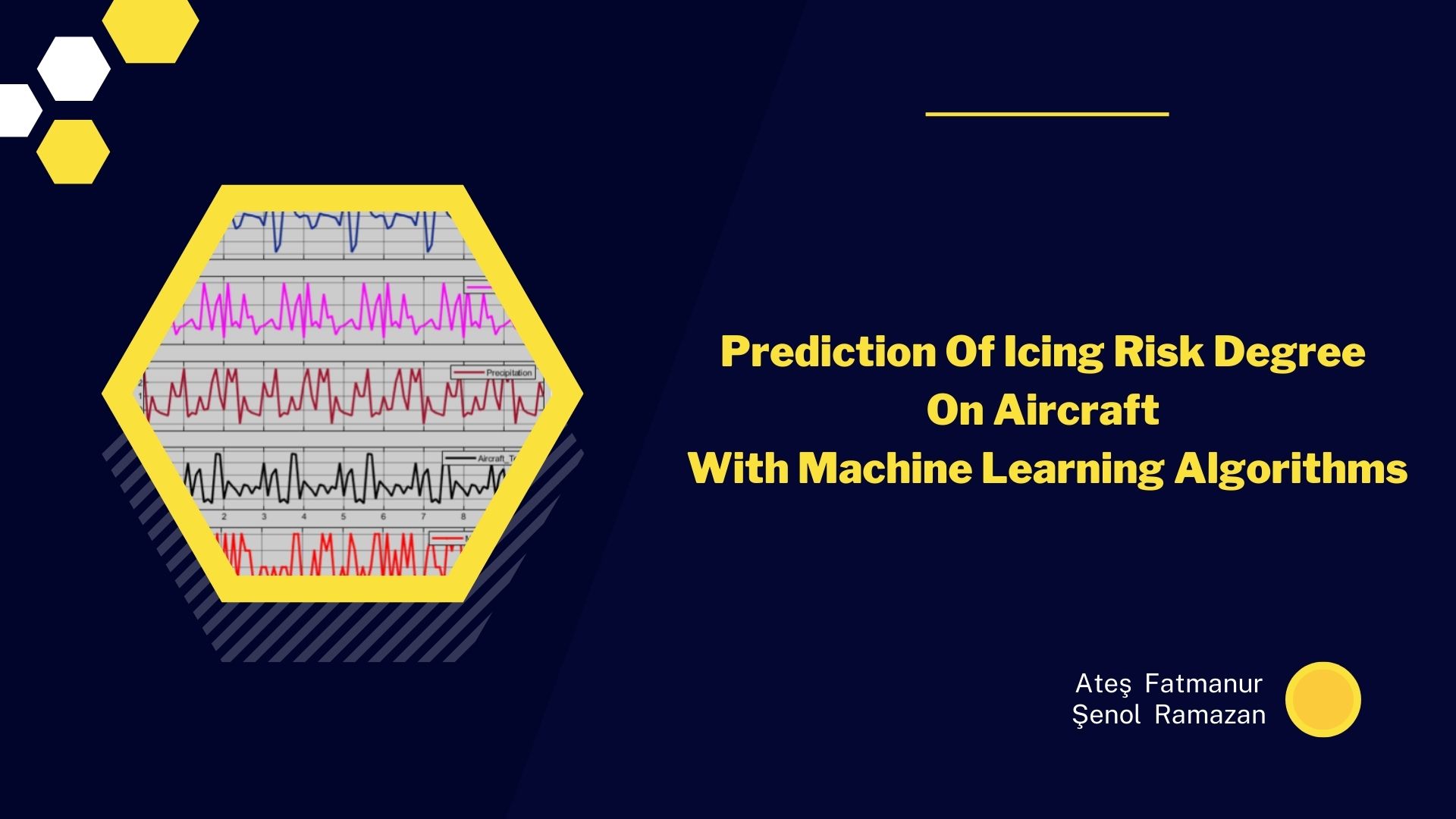
Abstract | Icing poses a threat for aircrafts, as it does for all other areas in general. From the past to present, many aviation accidents have occurred and are still occurring due to icing. Scientists have proposed several solutions to eliminate or minimize the problem of icing. To eliminate icing, de-icing systems have been introduced and anti-icing systems have been put into practice to prevent icing and these methods are presently being developed. However, these systems are generally put into use in aircrafts under pilot control. The pilot operates anti-icing and de-icing systems when she or he senses the presence of ice. The pilot's carelessness or lack of training then plays a major role in the occurrence of accidents. The study has tested seven different artificial intelligence algorithms for an aircraft that could lead to a direct operation of the de-icing/anti-icing system as a result of the estimated risk of ice. Simulink and Waijung blockset-supported modelling have been conducted and the diagram that will estimate the icing risk rating for different input values was embedded in the STM32F4 Discovery controller board and the results of the icing prediction were observed using RGB led. 88.59 percent value is obtained by the boosting algorithm for the optimum icing risk rating prediction. In conclusion, a model is proposed to ensure automatic activation of the anti-icing and de-icing systems in aircraft that will be used for predicting icing. |
Item Type | Research Article |
Title | Prediction Of Icing Risk Degree On Aircraft With Machine Learning Algorithms |
Author | Ateş Fatmanur Şenol Ramazan |
Institution | In Review |
Page | 17 |
Date | 2022-2-16 |
Language | English |
URL | https://assets.researchsquare.com/files/rs-1188167/v1/e705f2b1-dfd2-4ea4-bf06-510f36265ee1.pdf?c=1652538694 |
Library Catalog | DOI.org (Crossref) |
Tags | Icing on aircrafts, artificial intelligence, machine learning, supervised learning |
About author
Related Posts
-
EL802 Microprocessor SystemsLab 04 - Basic and Advanced PWM using STM32F4 MCU
-
Design Adaptive Fuzzy Sliding Mode Controller for Pantograph Mechanism Apply to Massage Therapy Robot for Healthcare
-
Model Based System Design of Conceptual Drive-by-Wire ECU Functions for Electric Vehicle Conversion
-
Analysis of Various PWM Techniques for Three-phase Asynchronous Motor
Related Products
-
Waijung 2 for ESP32As low as ฿4,500.00
Categories
Recent Posts
-
Aimagin IoT Solution
-
Aimagin Analytics KPI - แนะนำระบบติดตามและประเมินตัวชี้วัด (KPI)
-
Internet of Things (IoT) Feedback Control with Model-Based Design and Waijung 2 for ESP32 Workshop (Balancing robot controller project-based learning)
-
Internet of Things (IoT) Feedback Control with Model-Based Design and Waijung 2 for ESP32 Workshop (RC-circuit controller project-based learning)
-
Project 1B "Deploying Simulink and State flow to ESP32 using Waijung 2"
news
Aimagin Analytics
Job
Waijung
ESP32
Embedded System
Model-Based Design
Rayal Thai Navy
Aimagin
Introduction
Waijung2
Generation 2
Remote sensing
Artificial Intelligence
Review
Training Books
PID controller Design and analysis
Fuzzy Logic Controller
C Code
Temporal Table
Embedded Systems
Engineering Education
Microcontroller Board
Waijung Blockset
Induction Motor
Scalar Control
Speed control
STM32F4
Big Data
DC-DC converter
Hands-on power electronics education
Inverters
Mathematical model
Matlab
Microcontrollers
Waijung
Adders
Adjustable dc-link
Haedware
Harmonic analysis
orthogonal current decomposition control (OCDC)
power quality (PQ)
Reactive Power
Voltage control
voltage source converter (VSC)
Waijung block set
Journal Article
Waijung 1
STM32
Conference Paper
Clamps
CSVM
DSVM
FFT analysis
HSVM
Pulse width modulation
Support Vector machines
Swistches
Switching loss
swistching WAIJUNG
STM32
Journal Article
ESP32
DC motor
DC motors
Gears
Genetic Algorithm
Genetic algorithms
Genetics
Grey Wolf Optimization
PID controller
Position control
Steady-state
Ziegler Nichols
Boards
Gold
PV
Sensors
solar energy
solar tracking system
Sun
ARM Cortex M4
dc-dc converter
Hardware
Inverter
Logic gates
microcontroller
sine-PWM
Book
Waijung 1
Brain modeling
error regularization
Load modeling
normolized least mean square
Photovoltaic systems
Power quality
Power system harmonics
solay photovoltaic
Transient analysis
lower shoot-through
qZ-source T-type inverter
sixty-degree PWM
upper shoot-through
Waijung 2 for ESP32
IoT
Simulink
Webinar
WAijung 2
New Features
Generate Code
External Mode
Computer hardware and software
Drive-by-wire
Electric vehicles
Electronic control units
Simulators
Simulation and modelling
vehicle drivers
active disturbance rejection
adaptive control
solar tracking systems
trajectory tracking control
ไมโครคอนโทรลเลอร์
ระบบแบบอันดับที่หนึ่ง
วงจรตัวต้านทาน - ตัวเก็บประจุ
ไวจังบล็อคเซ็ต
ฮาร์ดแวร์ในวงลูป
ตัวควบคุมแบบพีไอดี
เทคนิคแบบฮาร์ดแวร์ในวงลูป
ระบบควบคุมอุณหภูมิ
Waijung Blockset
การควบคุมมอเตอร์
Simulink
Custom Code Block
STM32f407
Matlab
Microgrids
Attitude detenmination
IMU
MATLAB/Simulink
การหาค่าการวางตัว
Cylindrical-parabolic solar collector design and testing
GPS solar tracking
fuzzy logic-based solar tracking
solar renewable energy
Monitoring system
Medicine Storage
Internet
GSM
Wireless
สมองกลฝังตัว
ระบบตรวจวัด
ห้องเก็บยา
อินเตอร์เน็ต
ระบบโทรศัพท์จีเอสเอ็ม
ไร้สาย
HTTP Server Block
Web Server
Cascaded H-bridge
Induction motor drive
Multicarrier SPWM
Multilevel inverter
Speed control.
Diophantic
Integral Absolute Error (IAE)
Integral Time Absolute Error (ITAE)
Proportional-Integral and Derivative (PID)
Reference Signal Tracking (RST)
Proportional
Integral and Derivative (PID)
The phase-locked loop
grid-tied system
SOGI-FLL
HIL
CAN bus
Fuzzy controller
Brushless DC motor (BLDCM)
electric vehicle(EV)
Regenerative Braking
STM32F4 Discovery board
Waijung block set.
IT
Classic Controls Experiments
Low-Cost Hardware
Indoor 3D Reconstruction Using Camera
IMU and Ultrasonic Sensors
3D Point Cloud
Position Estimation
Iterative Closest Point (ICP)
Ultrasonic Sensors Distance Measurement
3D Indoor Reconstruction
DC – DC Converter
buck converter
Wind turbine
Emulator
Converter
MPPT
Tip speed ratio
กังหันลม
การจ าลอง
คอนเวอร์เตอร์
การควบคุมก าลังไฟฟ้าสูงสุด
อัตราส่วนความเร็วทิป
Multilevel Grid
Topology
MBD
sliding mode control
lyapunov stability
adaptive fuzzy control
chattering
temperature control unit
rapid control prototyping
MPC control strategy.
32-bit arm microcontroller
MATLAB Simulink
maximum power point technique(MPPT)
modified(DC-P&O)
PSIM1
Phase-locked Loop
Frequency Lock Loop
STM32F407VG Microcontroller
DC-Signal Cancellation Block
Waijung2 for ESP32
Speed Monitoring
Three-Phase Induction Motor
SPIFFS
Volatile Storage
PD Control
kinematic analysis
Simscape Multibody
3-DOF planar robotic manipulator
Bidirectional Converter Curcuit
PID Control
วงจรแปลงผันไฟฟ้ากระแสตรงแบบสองทิศทาง
การควบคุมแบบพีไอดี
LQR
balancing cube
Control theory
embedded systems
PWM
Icing on aircrafts
artificial intelligence
machine learning
supervised learning
ARM Cortex
Graphocal programming
Harmonic analysis
Power filters
active filter circuit
Power Electronics
Grid Converter
Embedded System
Identification
Particle Swarm Optimization
PI controller
การระบุแบบจำลองทางคณิตศาสตร์
มอเตอร์ไฟฟ้ากระแสตรง
วิธีกลุ่มอนุภาค
ตัวควบคุมพีไอดี
Senses Weizen
grid
OTSR
PIL
PMSG
wind
Microcontroller
Model Based Design
Rapid Prototyping
stability
variable structure systems
control system synthesis
augmented system
higher order sliding mode
system stability
dynamic sliding mode control
Mathworks
Data Analytics
#modelbaseddesign
#matlab
#simulink
XCP
#Waijung 2 for ESP32
#IoT
#AimaginAnalytics
#DataAnalytics
#Analytics
#Waijung2forESP32Challenge2023
#Waijung2forXilinxZynq-7000